June 13, 2024
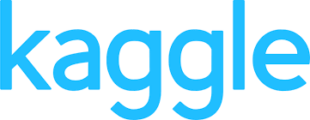
By: AEOP Membership Council Member Iishaan Inabathini
My first glance at Kaggle’s data science competitions thrilled me. I could register, compete and win thousands of dollars. Some competitions even invited winners to present at top conferences like NeurIPS. The accessibility hooked me. As a high school junior, I was spending my free time learning about machine learning and statistics because I enjoyed the content, but I had only applied my knowledge a few times outside of courses and textbooks. Kaggle was to be my Nemean lion – my first great success.
I chose the most challenging competition, featuring prizes of $100,000 and five invitations to NeurIPS. After reading about the data and evaluation metrics, I didn’t bother too much with Kaggle’s most valuable resource: expert advice. In my eagerness, there wasn’t much to do except download the data and get started with exploratory data analysis.
My entrance into Kaggle wasn’t what I expected. I might have been familiar with the theory, but after joining Kaggle, I learned terminology I haven’t heard anywhere else. Placement is decided by a number, so users employ several tricks to push for tiny improvements. Some users will post the predictions that earned them a high score, which other users will “blend” with their predictions. I’ve seen success from users who spent five minutes blending the predictions of other users.
I jumped into the lion’s cave without knowing anything about it, and I hadn’t yet developed the type of skills needed to figure things out “in the moment”. I fired my arrows, each bouncing off the lion’s coat, and it was too late before I realized what was happening. Everything took me much longer than it should have, and I quickly fell behind experienced users and those who took advantage of shared work.
Many experienced data scientists post their exploratory data analysis for beginners to see and learn from. Aside from competition success, Kaggle ranks users based on their shared notebooks, discussions and datasets. Experts share their great work while the competition is ongoing. Beginners and experts alike have public discussions about what they are working on, benefitting all competitors.
Here’s what I have learned from my experience:
Kaggle is focused purely on performance on specific datasets. Success can have very little to do with theory, and it is not uncommon to see success through the fine-tuning of pre-trained models.
Theory has always been the fascinating side of machine learning for me; there are probabilistic perspectives that are nothing short of enlightening. But Kaggle only cares about empirical results, and competitors don’t have the time to focus on anything more than a number. I didn’t truly understand what this meant until I saw how the final leaderboard played out; I saw some amazingly insightful work fall behind. I felt like someone had dumped a bucket of cold water on my warm and fuzzy theoretical comfort space, and honestly, it served as a fair warning about what commercial machine learning can look like.
A more comforting point of view: when I clicked through the discussions of some of Kaggle’s top competitors, I learned I wasn’t alone in bombing my first competition. My experience appears to be Kaggle’s universal truth.
If you’re interested in competing, take some time to explore the top submissions of large competitions. Look out for how the principled approach generally wins out (theory still has its place) and look for patterns. There’s a reason that Kaggle’s best consistently place high. Take advantage of Kaggle’s diverse resources: past competition submission writeups, computing power, datasets, notebooks, courses and people.
Find a Volunteering Opportunity
Visit our Program Volunteers page for a tool to find the best opportunity for you.
eCYBERMISSION Grants
The eCYBERMISSION Mini-Grant is intended to support teachers/program leaders as they implement eCYBERMISSION with their teams. Educators (formal and informal) of students in grades 6-9 are encouraged to apply. Special consideration is given to Title 1 schools and to those with underserved/ under-represented populations.